Observational Cosmology
Astrophysics
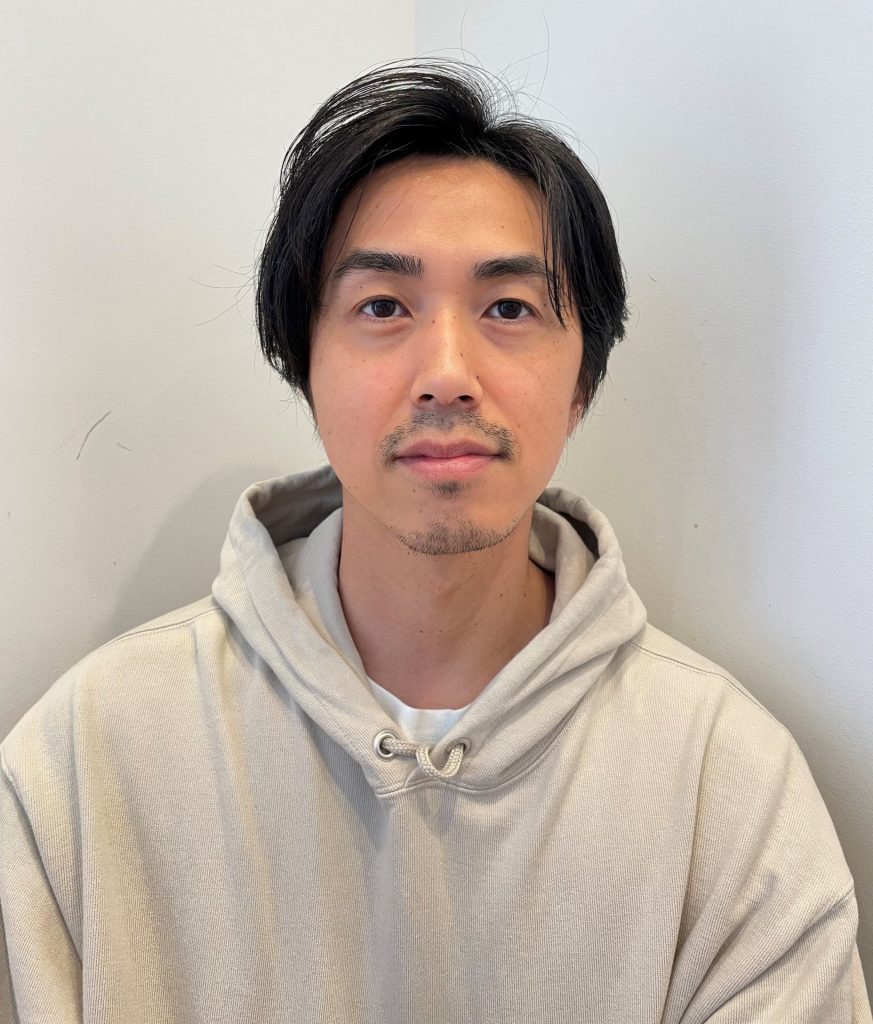
SHIRASAKI Masato[Affiliation April 2023-March 2024]
Starting year 2023
National Institutes of Natural Sciences, National Astronomical Observatory of Japan
Research Enhancement Strategy Office
Assistant Professor
Research fields
Research Interests
Large scale structure of the universe
Dark Matter
Accelerating expansion of the universe
N-body simulation
Data Science
Professional Memberships
Astronomical Society of Japan
Main research topics
Modern astronomers face two major mysteries in understanding the evolution of the universe.
These are: (1) invisible gravitating mass is necessary for the formation of observable galaxies in the universe, and (2) the universe has been expanding at an accelerated rate since about 7 billion years ago. The invisible matter is commonly called dark matter, and its existence cannot be explained within the framework of modern physics. In addition, the rate of expansion of the universe cannot accelerate when there is only universal gravitation in the universe. The accelerating expansion of the universe suggests that a repulsive force exists in the universe that opposes the universal gravitation, but it remains unclear what creates the repulsive force.
I have used numerical simulations and observational data to identify physical properties of dark matter and the accelerating expansion of the universe. In particular, I am interested in a phenomenon called gravitational lensing to map the ubiquitous distribution of cosmic matter, and using the statistical information in the map as a clue to tackle the two major unsolved problems in astronomy. Recently, I have been working on the refinement of maps that can be drawn by gravitational lensing effects, incorporating convolutional neural networks (CNNs), which have been making remarkable progress in the field of image analysis. Although the data so far have been related to astronomy, the CNN itself can be applied to research in various academic fields, not limited to astronomy. Their high flexibility is useful for building mathematical models to predict complex phenomena in nature. Through exchanges with T-GEx fellows, I will explore the possibility of collaborative research in fields other than astronomy.
Representative papers
Masato Shirasaki, Kana Moriwaki, Taira Oogi, Naoki Yoshida, Shiro Ikeda, Takahiro Nishimichi, “Noise reduction for weak lensing mass mapping: an application of generative adversarial networks to Subaru Hyper Suprime-Cam first-year data”, Monthly Notices of the Royal Astronomical Society, Volume 504, 2021, pp.1825-1839
Masato Shirasaki, “Probing Cosmic Dark Matter and Dark Energy with Weak Gravitational Lensing Statistics”, Springer Singapore, 2016, 136
Research URL
researchmap https://researchmap.jp/masato_shirasaki?lang=ja
Global issues to be solved through this project
Developing an efficient algorithm for transform between dark matter and real observables in the
universe
Dark matter does not emit light itself, but as a major source of gravity, it plays an important role in the formation of the structure of the universe. The spatial distribution of dark matter is determined by gravity and can be precisely predicted using simulations, whereas there is no established theory for stars and gas that can be detected by actual astronomical observations, making it difficult to accurately predict their distribution. We aim to develop an algorithm that can rapidly predict the spatial distribution of observables from the accurately predictable dark matter distribution using a semi-analytical model and machine learning. This algorithm will be important in extracting information on dark matter and the accelerating expansion of the universe from future multi-wavelength astronomical observations. In particular, uncertainties in modeling of the formation and evolution of stars and gas can be taken into account by changes of parameters in the semi-analytical model, enabling robust analysis in future observations.
Interview
No interview
News
No related news